In recent years, MICD and TU Delft have tested and refined the Crowd Safety Manager, together with other parties. This system provides insight into the crowds on the boulevard of Scheveningen (in the town of The Hague). Since it shows the flow of crowds, police and municipality are aware of when to intervene. However, it would be even better if the system were capable of anticipating. TU Delft scientist Serge Hoogendoorn and his team are developing models to predict crowds.
Project Predictor for the Crowd Safety Manager, January-December 2022
Team Serge Hoogendoorn, Panchamy Krishnakumari, Sascha Hoogendoorn-Lanser
What exactly is the purpose?
“The Crowd Safety Manager helps the municipality and police manage the crowds on Scheveningen’s boulevard: what tactical and operational measures should they take when? We were asked develop a model that can predict crowds in Scheveningen on top of that, based on weather forecasts combined with real-time and historical data. This involves both short-term forecasts, up to about two hours ahead, as well as longer-term forecasts, up to a week ahead.”
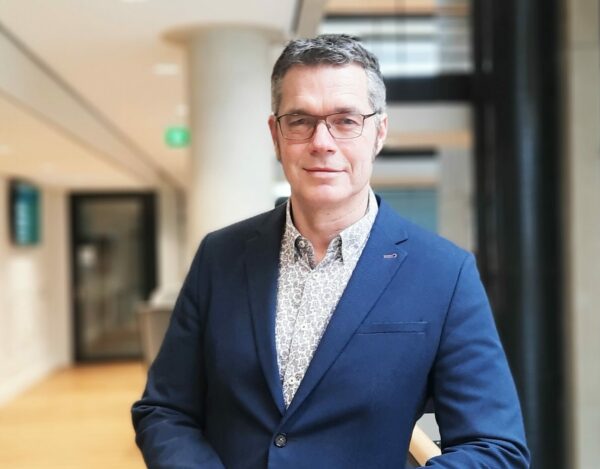
Prof. dr. ir. Serge Hoogendoorn, distinguished professor Smart Urban Mobility
The system already gives a good picture of the current situation. Why is it so important to be able to look ahead as well?
“In crowd control, managing large crowds, taking the right measures is as crucial as timing. Say, for example, you want to allocate extra enforcers, to keep the flow of pedestrians moving. It usually takes them at least 15 minutes to reach that spot. Then you’re always falling behind the curve. With a forecasting model, you are able to call the enforcers promptly and they will arrive on time. Obviously, these employees should be available when needed. Therefore, we want to look up to a week ahead, to make sure that enough staff are scheduled.
“However, an important factor is that human behaviour is difficult to predict, be it traffic jams or crowds. There are also convoluted relationships between the decisions of individuals and the eventual flows of people we observe. On top of the complexity of human behaviour, many other factors are of influence. From a scientific point of view, that actually makes it very interesting! With all the new data sources at our disposal, can we make sensible predictions? For instance, we hold data on the location of mobile phones, public transport, parking garage occupancy, measured traffic density on the street, as well as weather forecasts and even data from social media on the ‘sentiments’ of a crowd.”
Can you make sense of all that?
“It became quickly clear that we cannot easily incorporate all these different data types into traditional traffic engineering models. These are built by traffic engineers and hence contain ‘implicit traffic knowledge’, but they can’t handle information about the weather or sentiments for example. A completely different approach is modern AI, artificial intelligence. AI is perfect for recognising patterns in very diversified data, but it lacks traffic knowledge.
“So the idea popped up to create a combination of both. As we call it: ‘model-based meets data-driven’. We are basically applying AI technology that explicitly takes into account the behaviour of walking and traffic flows in networks and, simultaneously, learns which correlations can be distilled from the huge amounts of data. So the non-trivial correlations between crowds and factors of influence.
“The first results are very promising. We perceive that our approach has potential to deliver sufficiently accurate and reliable predictions. For both short- and long-term. Moreover, the underlying approach provides insight into the factors that determine the prediction of crowds. Think of week days or weekends, weather forecasts and specific events.”
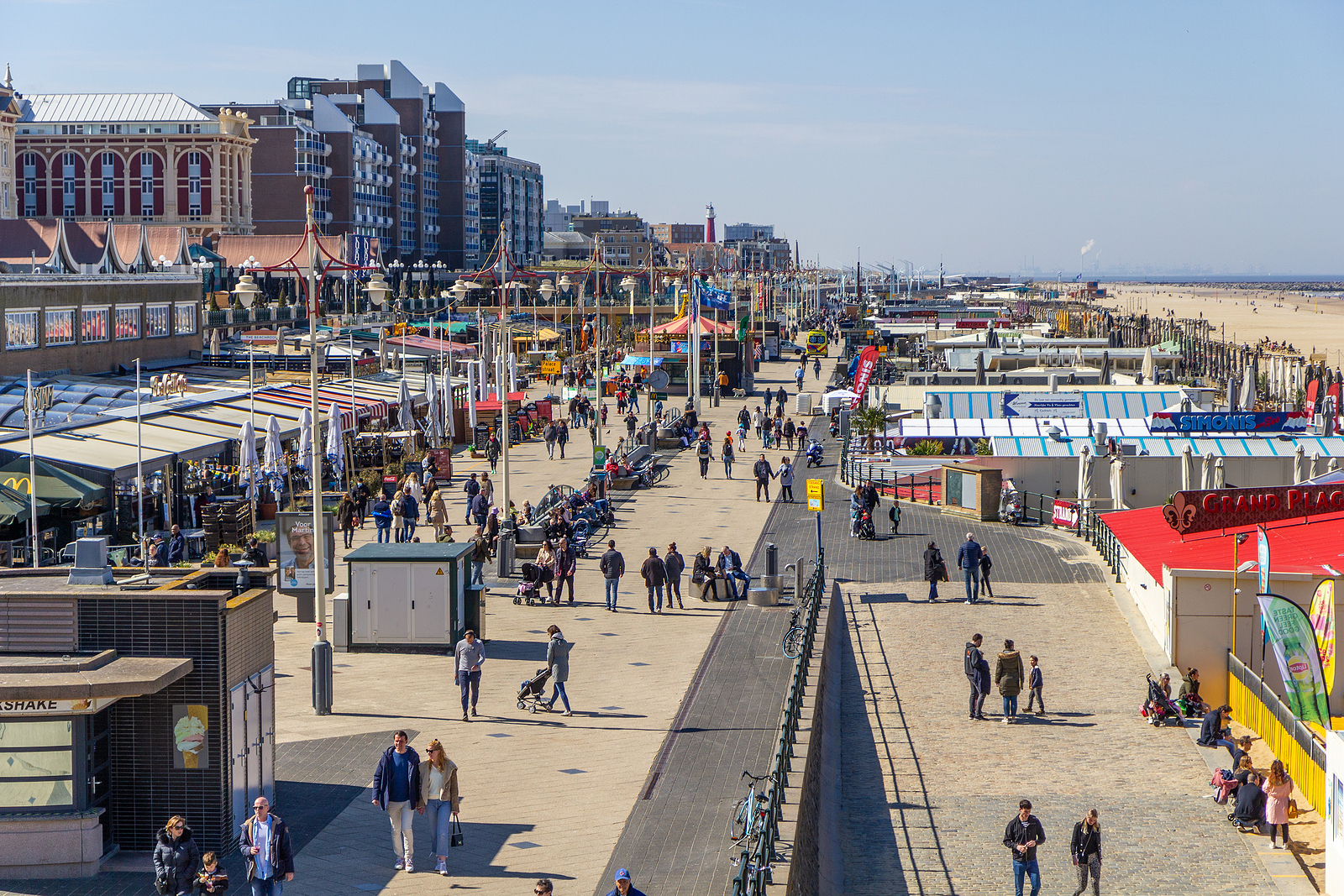
Nice! And how useful are the results of your experiments? Are they exclusively applicable on Scheveningen or are there more possibilities?
“The possibilities are definitely more extensive. Our approach is generic because the two main elements are generic. AI is widely applicable and traffic engineering knowledge is location-independent as well. So we expect our method to work in different locations and situations. To verify this, we are currently looking for other applications. This might be outdoors, such as a model for congestion in inner cities, as well as indoors: congestion in stations or educational buildings. I think the more types of conditions we can test, the better. An ordinary Saturday afternoon on a popular shopping street versus a Saturday afternoon with a demonstration on a square downtown Amsterdam, for example. That will help us further improve the methodology. Likewise for Scheveningen’s Crowd Safety Manager.”
When would you really consider the research successful?
“Our focus so far has primarily been on predicting crowds. I consider this experiment to be truly successful once we can convert the predicted crowd into a tangible risk for a calamity. To do so, understanding crowding alone is insufficient. We need to include ‘aggravating factors’ such as crowd sentiment, composition, the reasons why people are flocking here and so on. As a researcher, I preferably want to conduct trials in several locations and under various conditions. So we are not done with it yet!”
More information:
Crowd Safety Manager
AI for Mobility Lab